AI - Open or Closed for Business?
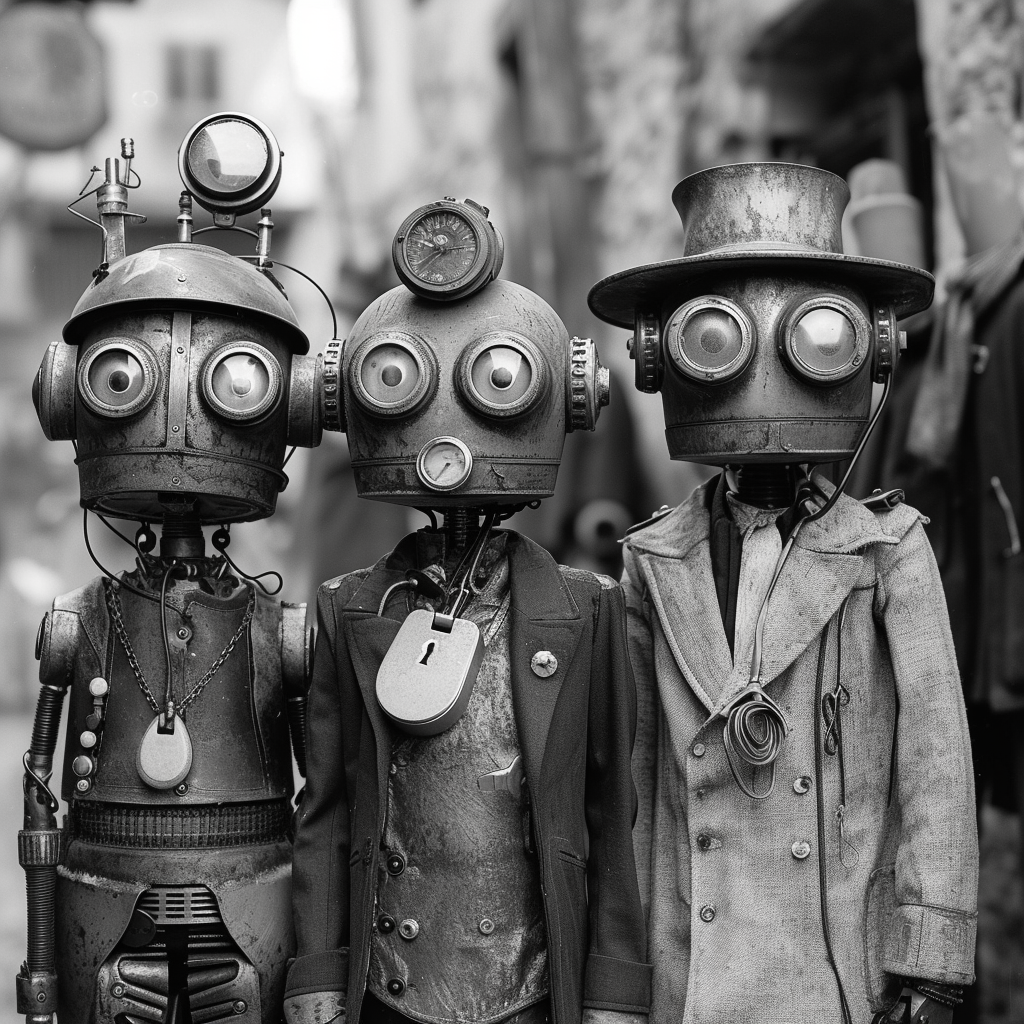
The scholarly publishing sector is locked up in a lot of proprietary systems. These systems are expensive whether the publisher built it themselves or they rent their workflow tools from a vendor. Numerous compelling reasons exist for publishers to wholeheartedly embrace open source, not the least of which is the substantial reduction in their technology-related expenses throughout their entire technology stack. However, my experience has been that there are a lot of publishers that do not understand what open source is or it's value to them.
While we are seeing encouraging forward movement on this issue I'd like to briefly discuss a new wave of open source that might bring publishers to open source with the possibility of accelerated adoption of open source: open source Language Learning Models (LLMs - I also refer to LLMs interchangeably as 'models' throughout the text).
Taxonomy of LLMs: Open, Fauxpen, Closed
To understand the value of open source LLMs, it's crucial to begin with a solid understanding of what 'open source' truly means. This comprehension is rooted in recognizing the three broad categories of licensing models that govern these technologies:
- Proprietary LLMs: These models provide public or enterprise services but withhold their source code. To leverage these models you must use the centralized services provided by the vendor. You are entirely beholden to their licensing terms, cost structures and practices. Prominent examples include ChatGPT and Claude.
- Open Source LLMs: These models offer fully accessible source code and licensing terms that grant unrestricted use, modification, and redistribution.
- Fauxpen Source LLMs: The providers of these models assert their open-source status, but beneath this claim lie concealed licensing restrictions, especially concerning commercial applications. This pretense of openness veils their departure from open-source licensing terms.
Lets look a little closer at each of these licensing offerings and what issues they raise for publishers.
Proprietary LLMs
Proprietary large language models (LLMs), which are the most well known, are developed and offered by leading organizations such as OpenAI, known for ChatGPT, and Anthropic, the creators of Claude. A proprietary (or 'closed') LLM provider does not offer the source code for you to examine or deploy in your own technical environment. Instead you must use the LLMs through the services offered by the vendor. This means you are beholden to their pricing model, terms and conditions, and you must submit your data to their systems for processing by the LLM.
There are several issues publishers are considering before using these models.
First up, many publishers are concerned (and some are turning to legal options to address) that these services have consumed their 'copy protected' material to train the systems. Not surprisingly, the technology providers disagree. OpenAI, for example, is taking the stance that consuming publisher data in training is fair use. In a written statement to the UK's House of Lords Communications and Digital Committee OpenAI has stated (https://www.theregister.com/2024/01/08/midjourney_openai_copyright/):
we believe that legally copyright law does not forbid training
https://committees.parliament.uk/writtenevidence/126981/pdf/
In other words, OpenAI believes the consumption of copyrighted material used to train the model to be covered by fair use.
training AI models using publicly available internet materials is fair use, as supported by long-standing and widely accepted precedents.
https://openai.com/blog/openai-and-journalism
Beyond those pursuing legal remedies, OpenAI's stance appears to have had a chilling effect on adoption among publishers influenced by broader concerns over the company's approach to intellectual property and AI. In my discussions with publishing service providers, some publishers seem hesitant to incorporate AI into their workflows—not because they wish to take legal action against OpenAI specifically, but because they view OpenAI's position as acting in bad faith towards creator rights and ownership.
There is a delicate dance for these publishers - hesitating to use AI on the principle that it may have been trained on copyrighted material could also be a strategic misstep. As AI continues to enhance competitiveness in the publishing industry, avoiding its use due to these concerns might put publishers at a disadvantage compared to those who embrace AI advancements.
However, there are also other concerns for publishers. As I wrote here the complexities surrounding data privacy of these proprietary LLMs present significant challenges. Many publishers are cautious about uploading data to large proprietary AI models due to privacy concerns and unclear data usage and storage policies. OpenAI's 'vision AI' documentation offers some reassurance, stating that images processed by their vision features are not retained for model training. However, contrasting statements in OpenAI's enterprise privacy policy suggest that data provided by users is used to 'enhance model accuracy'.
OpenAI trains its models in two stages. First, we learn from a large amount of data. Then, we use data from ChatGPT users and human trainers to make sure the outputs are safe and accurate and to improve their general capabilities
https://openai.com/enterprise-privacy
The apparent inconsistency creates ambiguity about how OpenAI manages user-provided data, leads to uncertainty and reluctance among publishers to adopt such technologies.
Another critical concern for publishers to contemplate (though not an exhaustive list) pertains to their potential liability when utilizing a proprietary Language Learning Model. Specifically, if your usage of a proprietary LLM is determined to infringe upon someone else's intellectual property, it could raise significant legal questions. Given that LLMs are trained on copyright-protected materials, can you be held liable for employing a service that has incorporated such content into its training data? Further, can AI generated content reproduce IP infringing content when you use it? According to Universal Music this is happening:
The music publishers’ complaint, filed in Tennessee, claims that Claude 2 can be prompted to distribute almost identical lyrics to songs like Katy Perry’s “Roar,” Gloria Gaynor’s “I Will Survive,” and the Rolling Stones’ “You Can’t Always Get What You Want.”
https://www.theverge.com/2023/10/19/23924100/universal-music-sue-anthropic-lyrics-copyright-katy-perry
Thad Mcllroy has written on these issues in Publishers Weekly.
It appears the large proprietary LLMs are very aware of this issue and some, like OpenAI, provide what is known as a 'copyright shield.
OpenAI’s indemnification obligations to API customers under the Agreement include any third party claim that Customer’s use or distribution of Output infringes a third party’s intellectual property right.
https://openai.com/policies/service-terms
OpenAI's service terms appear to provide indemnification to API and enterprise customers only against third-party claims of intellectual property right infringement resulting from the use of OpenAI's output. This might be enough for some publishers, however, this indemnity doesn't apply in several situations, such as when the customer knew or should have known the output was infringing, ignored or disabled citation or safety features provided by OpenAI, modified the output, or used it in combination with non-OpenAI products or services. This indicates that while OpenAI offers some protection, users need to be cautious and comply with specific guidelines to benefit from this indemnification. Of course, many publishers will be shy of this issue until this ground has been thoroughly worked out and tested in courts around the world.
Open Source LLMs
As discussed above, many publishers are wary of entrusting their content to services like OpenAI, driven by several legitimate concerns. These include the use of their content for training purposes, potential privacy issues, potential liability and possibly other sundry issues such as the risk of data leaks.
In this context, adopting open source Language Learning Models (LLMs) presents a significant strategic benefit for publishers. This approach allows them to bypass the need to share copyrighted content with external AI services, directly addressing key content security concerns. By setting up these LLMs in their own controlled hosting environments, publishers lock the systems down while gaining the ability to integrate them into both internal and customer-facing systems.
Furthermore, the latest open source LLMs have achieved performance levels that are comparable to, or almost match, those of larger proprietary models, thus positioning themselves as a practical and reliable choice for enterprise use.
Open source LLMs are not only secure (if you take care of the hosting environment) but also a technically competitive option for publishers, enabling them to leverage the full potential of AI while safeguarding their content and operational interests.
However, while there are significant advantages for publishers to use an open source LLM there are still some issues to be considered. There is, of course, the question of cost. Is deploying a open source LLM going to be cheaper than using a proprietary service? This will require cost analysis on a case by case basis. In general however, this will prove to be true at larger scales. Small publishers, and I am especially thinking of the very small Diamond AI multitude, will only find this to be the case if they have access to resources like volunteers, funding, or pooled resources to install and run open source LLMs. On the other hand, Open Access publishers may also ask themselves whether they need to care about data privacy issues at all as their content will be licensed through creative commons (although there might be other data they don't wish to share such as reviewer names etc).
It will also be a concern for some publishers that open source LLMs may have been trained on copyright protected material also. Unfortunately some technology providers are trying to obscure this issue. If we look at Metas LLama 2 website for example (Meta claims this is an open source LLM - see below on this problematic claim), we see statements like "Llama 2 was pretrained on publicly available online data sources." 'Publicly available' is a smoke and mirrors term that tries to dodge the IP issues - it doesn't mean the content wasn't 'copy right protected' when consumed for training purposes.
Further, if you use an open source LLM and the output does infringe someones copyright, you have no copyright shield protection (no matter how slim the offering by folks like OpenAI, it is better than no protection).
A last issue for publishers is that tracking the Open source LLM landscape is increasingly difficult because it is evolving so fast, however there are some lists evolving online to help organizations find bona fide open source LLMs. However, it stands to reason these efforts probably need to be increased and formulated to better assist the publishing sector in navigating this area.
Fauxpen Source LLMs
'Fauxpen source' (false-open source) refers to a practice where organizations or projects claim to be open source but don't fully adhere to its core principles or, indeed, it's licensing. Often this is done by organizations that perceive a positive brand value in their sector for being aligned with open source.
The case of publishing, however, utilizing a fauxpen LLM's could turn out to be a lot more serious than mere brand integrity if not considered carefully.
A recent high profile example of a fauxpen source LLM is Meta's LLaMa 2 which has adopted a 'open source alignment' marketing strategy. By labeling LLaMa 2 as 'open source,' Meta potentially attracts developers interested in innovating and improving upon their code while also appealing to enterprise sectors (eg Publishing) cautious of entrusting their data to shared models like those offered by OpenAI or Claude. It is a valuable market niche afforded to it by a claim to be open source.
However, the Open Source Initiative (OSI) has expressed concerns over Meta's LLaMa 2 licensing, which doesn't fully align with the standard Open Source Definition. The primary issue is the license's restrictions on certain types of commercial use and specific applications. The Open Source Definition requires free redistribution and use for any purpose, including commercial endeavors. This misalignment highlights the gap between Meta's claims of open-source and the foundational principles and requirements of open-source licensing.
The real-world implications of 'fauxpen source' claims, like those made by Meta, extend beyond mere brand enhancement. Publishers and developers who uncritically accept these open-source claims risk legal exposure if they use such technology. Unintentional violations of licensing terms can occur, especially when the full extent of the restrictions isn't understood. This can result in legal complications for users who believed they were compliant but were, in fact, breaching the license.
Thoughts from an Open Advocate
The terrain is difficult to navigate as there are so many issues to consider and many of these issues are in flux, working themselves out in court or in the minds of publishers everywhere.
I thoroughly believe open source LLMs are the way to go and offer immense value for publishers, however there are some ironies I can't help but sit back and ponder. What is the state of an open source LLM if it has been trained on publisher materials? As a staunch advocate for free content and open source principles, I find myself grappling with a fundamental question (legal issues aside): Is it ethically sound, in principle, for open source technologies to incorporate 'non-open' content as a foundational component in the development of these systems? My unease regarding this matter stems from a profound consideration of the very ethos and values that open source represents.
I am not alone in this thinking it seems as 'Fairly Training' is a new not-for-profit established to certify models that use only materials that are creative commons licensed or where the creators have given consent.
Whether or not there is a legal issue, and whether or not I as a user of an open source LLM I might be liable for infringing someone else's IP, the issue of IP is a concern. Notably however, these issues apply to all LLMs.
It seems it is hard to be a vegan when it come to open ethics and LLMs - sooner or later you are going to eat something proprietary.
My response to all this is very pragmatic - do I use LLMs or not? I can easily answer that for myself - I will (and do) use LLMs. The upside is too great and the downside of not using them is also significant. Given this no-win scenario I'll choose the less conflicted solution - open source LLMs.
In my opinion, publishers should also use open source LLMs for the same reasons.
Ironically, open access publishers may have 'less to lose' when it comes to data privacy issues and LLMs (unless we start factoring in concerns about scooping or user data). However, technology isn't just a tool, it is also a politic. If you are beholden to someones else's technology, and tied to them by licensing requirements and other legal constraints, you have effectively given way a lot of your autonomy, independence, and control of your future. This is why open source exists in the first place - to enable you to take back your tools. If someone else owns and controls the terms of your tools serious issues may emerge which you may dismiss now but come to be problematic down the road. By then it might be too late. We only need to look at the history of proprietary technologies in publishing to understand the significant downside of lock-in, insane license costs, your workflow being determined by the vendor, and waking up with your technology stack being owned by your competitor. I'm all for LLMs in publishing and I am completely for open source LLMs as the first choice.
A need for group effort
There are a lot of challenges here, even for open source LLMs. To address these challenges effectively, publishers may require a collective effort. Establishing an industry-wide initiative aimed at assessing and reporting on the openness of various AI technologies could prove invaluable. This group could begin by creating a matrix that explicitly outlines the intellectual property (IP) aspects of each model, helping publishers make informed decisions.
Such an initiative could evolve to become a pivotal advocate for responsible AI innovation with all types of technology providers (open or closed), fostering clear standards and facilitating candid dialogue between tech innovators and the publishing industry. While committees may not be everyone's favorite, they might offer a pragmatic means of ensuring publishers are part of the conversation rather than remaining passive observers on the sidelines.
In conclusion, the growing significance of open source LLMs for publishers necessitates a proactive approach. As publishers grapple with the evolving landscape of AI, establishing a shared industry group and matrix for evaluating AI technologies may be the key to ensuring responsible innovation and a brighter future for both publishers and technology innovators.
But in the end, I thoroughly believe the most sensible, and necessary, choice for publishers are open source LLMs.
© Adam Hyde, 2024, CC-BY-SA
Image public domain, created by MidJourney from prompts by Adam.
Member discussion